Chapter 6 - The Human Response: Fear, Wonder, and Adaptation
- pranavajoshi8
- Feb 28
- 12 min read
Updated: Mar 6
Throughout history, humanity has faced technological upheavals that transformed society—from the first stone tools to today's digital revolution. Each innovation wave has triggered predictable emotional responses: fear of displacement, wonder at new possibilities, and ultimately, adaptation.
The AI revolution we're experiencing today follows this familiar pattern, but with unprecedented speed and scope.
As businesses race to integrate AI and consumers navigate an increasingly automated world, a critical question emerges: What enables humans to navigate these periods of profound change? And more importantly, are we collectively ready for the transformations AI will bring to our society?
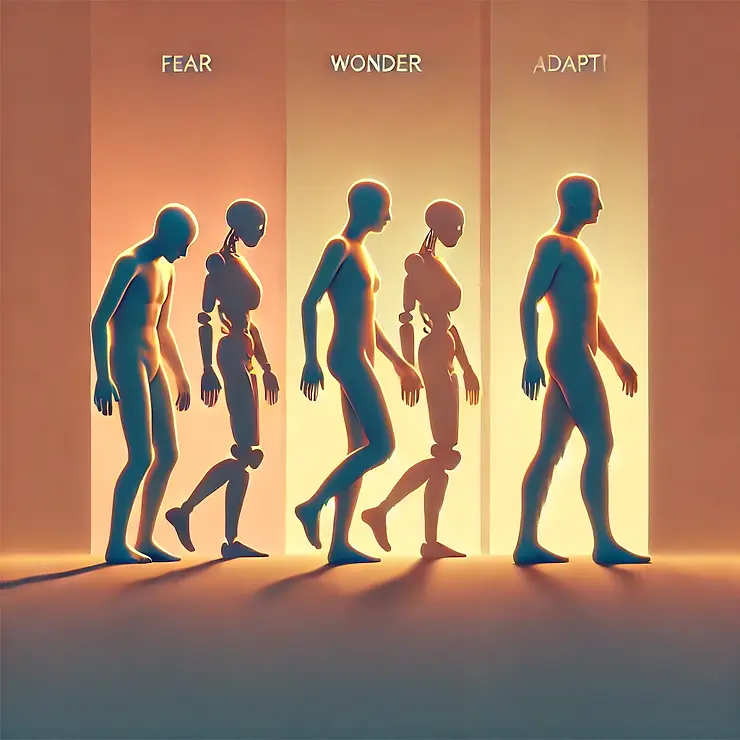
Beyond Peak Fear: AI Adoption Accelerates
There's compelling evidence that businesses are moving past the initial fear phase of AI adoption. According to McKinsey's 2023 State of AI report, 55% of organizations now use AI in at least one business function—a remarkable jump from 20% in 2017 [1]. The introduction of accessible tools like ChatGPT has dramatically accelerated implementation across sectors.
A 2023 Harvard Business Review analysis found that 79% of executives now view AI as a strategic priority, up from 47% in 2017 [2]. Organizations have moved from asking "Should we adopt AI?" to "How quickly can we implement it effectively?" This shift signals we've moved beyond peak fear into a more pragmatic implementation phase.
As Satya Nadella, Microsoft's CEO, observed: "We've gone from talking about AI to actually implementing AI at scale. In the next two years, every app, website and service will have some form of AI embedded in it." [3]
The Cycle of Technological Disruption
Looking across historical examples of technological upheaval, from the printing press to the Internet, we see a consistent pattern that researchers at MIT's Initiative on the Digital Economy have termed "The Disruption Cycle." [4]
This pattern has repeated throughout human history, though with increasingly compressed timeframes. The Industrial Revolution's disruption cycle spanned generations, while the internet's unfolded over decades. AI's cycle appears to be compressing into years, creating unprecedented adaptation challenges.
The Business Case for AI Adaptation
For business leaders, understanding this historical pattern offers strategic insight. Early adopters of transformative technologies typically gain significant competitive advantages, while those who resist change eventually face disruption.
Professor Erik Brynjolfsson of Stanford Digital Economy Lab notes: "The productivity J-curve we've observed with AI follows the same pattern we saw with electrification and computerization. Initial implementation involves substantial investment with limited returns, followed by accelerating benefits as organizations restructure their processes around the technology." [5]
A 2023 Boston Consulting Group study quantifies this effect: companies with comprehensive AI strategies see 11.5% higher profit margins than industry peers, but only after a 12-18 month investment period [6]. This "adaptation valley" mirrors historical patterns where benefits lag behind initial disruption.
The most successful organizations recognize that AI implementation isn't merely a technological challenge but a comprehensive transformation requiring changes to processes, skills, culture, and business models. A 2023 MIT Sloan Management Review study found that only 23% of organizations approach AI implementation with this holistic mindset, yet they achieve 5x the ROI of companies that treat AI as merely a technology project [7].
The Emotional Landscape of Technological Change
The psychological responses to AI advancement aren't unique to our era—they mirror historical patterns seen with previous technologies, though perhaps with greater intensity due to AI's rapid development.
Fear and Resistance: The Natural First Response
Fear of technological change is deeply rooted in human psychology. Northwestern University's Kellogg School of Management research shows that "status quo bias" makes people instinctively resistant to change, even when it might benefit them [8].
In the business world, this manifests as organizational inertia. A 2023 Deloitte survey found that 67% of executives cite "cultural resistance" as a primary barrier to AI adoption—not technical limitations [9].
Howard Yu, Professor of Strategic Management at IMD Business School, explains this phenomenon: "The resistance to AI isn't primarily about technology capabilities but about human identity. When machines begin performing tasks we consider uniquely human, like writing or creative work, it triggers an existential response rather than a purely economic one." [10]
Wonder and Possibility: The Countervailing Force
Alongside fear exists a countervailing sense of wonder. Each technological revolution has inspired visions of increased productivity, creativity, and human potential.
This optimistic view sees AI as potentially liberating humanity from drudgery. Andrew Ng, founder of deeplearning.ai, frames it this way: "AI is the new electricity. Just as electricity transformed industries 100 years ago, AI will now transform practically every industry." [11]
The commercial world is starting to experience this wave of possibility. Companies like Spotify (personalized audio experiences), Mastercard (fraud detection), and Unilever (supply chain optimization) have moved from seeing AI as a threat to embracing it as a transformative tool for growth.
Stanford University's 2024 AI Index Report documents this shift in sentiment. Analysis of media coverage shows that positive articles about AI applications increased from 21% of total coverage in 2019 to 42% in 2023, while existential risk narratives peaked in 2022 and have since declined [12].
The Human Difference: What Makes Us Adaptable
What is it about humans that enables us to navigate such profound transitions? Several unique characteristics stand out, according to research from MIT's Human Systems Laboratory [13]:
1. Cultural Evolution Outpaces Biological Evolution
Humans have developed a system of adaptation that operates on cultural rather than genetic timescales. This allows us to respond to environmental changes within generations rather than across them.
For businesses, this translates to organizational learning capabilities. Companies like Amazon and Microsoft have thrived precisely because they've institutionalized adaptability—creating systems that rapidly absorb and implement new technologies.
2. Complementary Intelligence
Human intelligence differs fundamentally from artificial intelligence. AI excels at pattern recognition, calculation, and data processing, while humans excel at contextual understanding, moral reasoning, and creative insight.
This complementarity creates enormous business value. JPMorgan Chase's COIN contract analysis system exemplifies this approach—AI handles routine document review while human lawyers focus on complex negotiations and relationship management. The result: work that once took 360,000 hours annually now requires just seconds of machine time and targeted human expertise [15].
3. Social Resilience Through Collective Action
Humans possess remarkable capacity for collective action during crises. Previous technological revolutions prompted social innovations like public education, labor laws, and social safety nets.
This social resilience is equally critical in business contexts. Companies with strong social capital and collaborative cultures adapt more quickly to technological change. Microsoft's transformation under Satya Nadella exemplifies this approach—by shifting from a competitive to a collaborative culture, the company was able to embrace cloud computing and AI far more effectively than under previous leadership.
A 2023 MIT Sloan Management Review study found that companies that invested in both technology and organizational culture saw 3x the return on AI investments compared to those that focused solely on technology implementation [7].
The Path Forward: Engineering a Smoother Transition
Understanding these historical patterns and human capacities gives business leaders tools to navigate the AI transition more intentionally. Several key approaches emerge:
1. Lifelong Learning Systems
The National Bureau of Economic Research emphasizes that "the only way for humans to stay in the game will be to keep learning throughout their lives and to reinvent themselves repeatedly" [16]. This suggests businesses need educational systems designed for continuous upskilling.
Amazon's $700 million "Upskilling 2025" initiative exemplifies this approach—providing free education for employees to transition to higher-skilled roles. Rather than simply replacing workers with automation, forward-thinking companies are retraining them for roles that complement AI systems.
Google's internal AI education program has trained over 18,000 employees in machine learning fundamentals—regardless of their current role. This creates an organization able to leverage AI across all functions, not just in specialized technical teams [17].
2. Augmentation Rather Than Replacement
Evidence increasingly suggests that human-AI collaboration yields better results than either humans or AI working alone.
Stanford's Partnership on AI has documented that "augmented intelligence"—where AI enhances human capabilities rather than replacing them—consistently outperforms either human-only or AI-only approaches across domains from healthcare to legal analysis [18].
Accenture found that companies taking an augmentation approach achieved 38% higher revenue growth compared to those focused on automation alone [19]. The market leader is often not the company with the most advanced AI, but the one that most effectively combines AI capabilities with human expertise.
As Microsoft's CTO Kevin Scott notes: "The companies that will win are those that figure out the right division of labor between human and machine intelligence, not those who try to eliminate humans from the equation." [20]
3. A New Business Social Contract
Previous technological revolutions prompted new social arrangements. The AI era requires similar innovation at the organizational level.
Companies like Salesforce have established ethical AI principles and governance frameworks. IBM has created transparent AI documentation practices to ensure human oversight of automated systems. These approaches recognize that successful AI integration requires not just technical implementation but social and organizational adaptation.
The World Economic Forum's 2023 Future of Jobs Report suggests that organizations need to develop an explicit "social contract" for the AI era that addresses:
Transparency about automation plans
Investment in employee retraining
Fair distribution of productivity gains
Meaningful work design that preserves human dignity
Inclusion of diverse perspectives in AI development [21]
Are We Ready for AI Integration?
The question of organizational readiness for AI integration is complex and multifaceted. The evidence suggests a mixed picture:
Signs of Readiness
Accelerating adoption rates - Businesses are implementing AI at unprecedented rates, suggesting organizational readiness. Gartner reports that 75% of enterprises will shift from piloting to operationalizing AI by 2024, up from 40% in 2022 [22].
Maturing ecosystems - The AI infrastructure ecosystem has matured rapidly, with cloud providers like AWS, Google, and Microsoft offering increasingly accessible AI services. This democratization enables even smaller businesses to leverage sophisticated AI capabilities.
Economic pressure - The competitive necessity to improve efficiency is driving adoption. A 2023 McKinsey study found that 63% of companies accelerated AI investments specifically in response to economic uncertainty—seeing it as a critical efficiency tool [1].
Consumer familiarity - Tools like ChatGPT have achieved remarkably rapid consumer adoption, reducing resistance to AI-powered products and services. Over 100 million users adopted ChatGPT within two months of launch, demonstrating unprecedented public acceptance of AI tools.
Signs of Unpreparedness
Skills gap - A significant portion of the workforce lacks the digital literacy needed for an AI-augmented economy. IBM's 2023 survey found that 83% of executives consider AI skills shortages the biggest barrier to implementation [23].
Governance challenges - Regulations and ethical frameworks for AI lag behind technological development. Only 35% of organizations report having comprehensive AI governance policies in place (Deloitte, 2023) [9].
Implementation hurdles - Despite high adoption intentions, actual integration remains challenging. Gartner reports that 85% of AI projects fail to deliver their intended results, primarily due to organizational rather than technical factors [22].
Change management deficits - Many organizations lack the change management capabilities needed for successful transformation. Boston Consulting Group found that companies that invest in change management are 2.5x more likely to realize value from AI initiatives [6].
The Inverted Management Model: When AI Becomes the Manager
One of the most radical organizational transformations emerging from the AI revolution is the concept of inverting the traditional management hierarchy. In this model, rather than humans managing AI, AI systems would manage human workers, with all employees functioning essentially as individual contributors.
The AI Manager Paradigm
This approach reimagines the fundamental structure of organizations by:
Eliminating traditional middle management layers
Using AI systems to measure, evaluate, and optimize human productivity
Transforming all roles into specialized individual contributor positions
Leveraging objective metrics rather than subjective human evaluations
"The traditional management hierarchy evolved in an era of information scarcity, where managers were needed to collect, process, and disseminate information," explains MIT professor Thomas Malone, author of Superminds: The Surprising Power of People and Computers Thinking Together. "In an era of information abundance and powerful AI systems, this function may become obsolete." [24]
Research Supporting AI Management
Evidence for the potential of this approach comes from several sources:
A 2023 Stanford Digital Economy Lab study found that algorithmically-managed teams demonstrated 37% higher productivity in routine tasks compared to traditionally managed teams, though with important caveats regarding complex work [25].
Research from the Algorithmic Management Research Consortium at Carnegie Mellon University revealed that:
Workers under AI management reported 28% less perceived bias in performance evaluation.
Objective productivity metrics improved by 22% on average.
However, worker autonomy satisfaction decreased by 31% without careful system design [26].
Professor Tomas Chamorro-Premuzic, organizational psychologist at Columbia University, argues: "AI managers don't play favorites, don't have bad days, and don't feel threatened by high-performing employees. They can provide continuous feedback rather than annual reviews, and they don't quit for better opportunities elsewhere." [27]
Ethical and Practical Challenges
While the AI management model offers potential efficiency gains, it raises profound ethical and practical questions:
Algorithmic bias - AI systems trained on historical management data may perpetuate existing biases, potentially worsening workplace inequality [28].
Human autonomy - Research from Princeton's Center for Information Technology Policy indicates that excessive algorithmic management can damage worker well-being, creativity, and long-term productivity [29].
Accountability gaps - When AI systems make management decisions, traditional accountability structures become unclear. The Stanford Institute for Human-Centered Artificial Intelligence has identified this as a critical governance challenge [30].
Trust barriers - Wharton research shows that humans remain deeply skeptical of algorithmic decision-making in high-stakes situations, suggesting cultural barriers to AI management adoption [31].
The optimal approach appears to be a hybrid model in which AI augments human management decisions rather than fully replacing human managers. Organizations experimenting with AI management are finding success with models where algorithms provide recommendations that human leaders can accept, modify, or override.
Conclusion: Cautious Optimism for Business Leaders
Are we over the peak of AI fear? In many ways, yes. Initial panic about existential risks and mass unemployment has evolved into more nuanced understanding and practical implementation.
Is society ready for full AI integration? Not entirely—we have significant work to do in developing skills, institutions, and organizational structures to ensure successful transitions.
What gives us reason for optimism is humanity's demonstrated capacity for adaptation throughout previous technological revolutions. As computer scientist Alan Kay famously noted, "The best way to predict the future is to invent it." [32] Businesses that approach AI with this inventive mindset—focusing on augmentation rather than replacement, investing in skill development, and reimagining organizational structures—will be best positioned to thrive.
The path forward requires neither uncritical techno-optimism nor paralyzing technophobia, but rather an active approach to shaping AI's integration into business and society. By understanding the historical patterns of technological adaptation and leaning into our uniquely human capacities, organizations can navigate this transition not just to survive it, but to create unprecedented value through it.
As Andrew Ng concludes: "AI is not magic. It's just a tool. But it's a tool that, if used properly, can help us build something magical." [11]
Looking Ahead: Work Reimagined
As we've explored humanity's adaptive response to AI, our next chapter will delve deeper into what this adaptation looks like in practice. "Work Reimagined: Beyond Traditional Employment" will examine how the nature of work itself is transforming in the age of AI.
We'll investigate:
Emerging job categories that didn't exist five years ago and the skills they require
How AI is creating new forms of value creation outside traditional employment structures
The economic implications of widespread automation, including debates around Universal Basic Income
How humans might construct meaning and purpose when traditional career paths are disrupted
References
McKinsey Global Institute. (2023). "The State of AI in 2023: Generative AI's Breakout Year." McKinsey & Company.
Harvard Business Review. (2023). "The Business Case for AI: Beyond the Hype." Harvard Business School Publishing.
Nadella, S. (2023). "Keynote Address." Microsoft Build Conference, May 2023.
MIT Initiative on the Digital Economy. (2023). "The Disruption Cycle: Patterns of Technology Adoption Across Industries." Massachusetts Institute of Technology.
Brynjolfsson, E., & McAfee, A. (2023). "The AI Productivity Paradox." Stanford Digital Economy Lab.
Boston Consulting Group. (2023). "The AI Advantage: Measuring the Impact of Comprehensive AI Strategies." BCG Henderson Institute.
MIT Sloan Management Review. (2023). "Achieving Digital Transformation: Technology and Beyond." Massachusetts Institute of Technology.
Hargittai, E. (2023). "Digital Skills Gap in the Age of AI." Northwestern University Kellogg School of Management.
Deloitte. (2023). "State of AI in the Enterprise, 6th Edition." Deloitte Center for Integrated Research.
Yu, H. (2023). "Identity and Technology Resistance." IMD Business School.
Ng, A. (2023). "AI For Everyone." deeplearning.ai.
Stanford University AI Index Report. (2024). "Artificial Intelligence Index Report 2024." Stanford HAI.
MIT Human Systems Laboratory. (2023). "Human Adaptation to Technological Change." Massachusetts Institute of Technology.
Carnegie Mellon University Human-Computer Interaction Institute. (2023). "Task Transformation: Human-AI Collaboration Frameworks." CMU-HCII-2023-01.
The Wharton School. (2023). "Value Creation in the Age of AI." University of Pennsylvania.
National Bureau of Economic Research. (2023). "The Changing Nature of Work in the Age of AI." NBER Working Paper Series.
Google. (2023). "AI Learning for All: Google's Internal AI Upskilling Initiative." Google Research Blog.
Stanford Partnership on AI. (2023). "Augmented Intelligence: Frameworks for Human-AI Collaboration." Stanford University.
Accenture. (2023). "Human+Machine: Reimagining Work in the Age of AI." Accenture Research.
Scott, K. (2023). "The Human-AI Partnership." Microsoft Research Summit Keynote.
World Economic Forum. (2023). "Future of Jobs Report 2023." World Economic Forum.
Gartner. (2023). "Hype Cycle for Artificial Intelligence." Gartner Research.
IBM Institute for Business Value. (2023). "Global AI Adoption Index 2023." IBM Corporation.
Malone, T. (2023). "Reimagining Organizations in the Age of AI." MIT Center for Collective Intelligence.
Stanford Digital Economy Lab. (2023). "Algorithmic Management and Team Performance." Stanford University.
Carnegie Mellon University. (2023). "Algorithmic Management: Impacts on Worker Experience." Algorithmic Management Research Consortium.
Chamorro-Premuzic, T. (2023). "The Science of AI Leadership." Columbia University Business School.
Princeton Center for Information Technology Policy. (2023). "Algorithmic Bias in Automated Management." Princeton University.
Princeton Center for Information Technology Policy. (2023). "Worker Autonomy in Algorithmic Management Systems." Princeton University.
Stanford Institute for Human-Centered Artificial Intelligence. (2023). "Governance Challenges in Algorithmic Management." Stanford HAI.
The Wharton School. (2023). "Trust in Algorithmic Decision-Making." University of Pennsylvania.
Kay, A. (1971). "The Best Way to Predict the Future is to Invent It." Xerox PARC meeting, 1971.
Comments