Chapter 7 - Work Reimagined: Beyond Traditional Employment
- pranavajoshi8
- Feb 28
- 26 min read
Updated: Mar 6
The Great Work Transformation: A Historical Inflection Point
The relationship between humans and work stands at a pivotal inflection point. Throughout history, technological revolutions have transformed not just how we work, but what we consider work at all. The AI revolution represents perhaps the most profound shift yet—one that challenges our fundamental understanding of human productivity, creativity, and purpose.
The AI revolution we're experiencing today follows this familiar pattern, but with unprecedented speed and scope.
As Microsoft CEO Satya Nadella observes, "AI will fundamentally change every software category and business process. The question isn't whether AI will transform your work, but how you'll transform your work with AI" [1].
This transformation extends beyond simply automating existing tasks; it reconceptualizes the very nature of jobs, careers, and value creation. In this exploration, we'll examine not just what jobs might be created or eliminated, but how the substance of work itself is being reimagined.
The AI Revolution: Quantifying the Transformation
The World Economic Forum's Future of Jobs Report 2025 provides a comprehensive outlook on the evolving global labor market, focusing on the impact of technological advancements, particularly AI and robotics. The report projects a net increase of 78 million jobs by 2030, resulting from 170 million new jobs created and 92 million jobs displaced [2].
This transformation is largely driven by technological changes, with broadening digital access expected to be the most transformative trend, as 60% of employers anticipate it will transform their business by 2030.
Key findings from the report include:
Nearly 40% of job skills are expected to change, with 63% of employers citing the skills gap as their primary challenge in business transformation.
The three fastest-growing jobs in percentage terms are big data specialists, fintech engineers, and AI and machine learning specialists.
86% of respondents expect AI and information processing technologies to transform their business by 2030.
The green transition and demographic shifts are generally expected to create additional jobs, while economic uncertainty is expected to displace jobs.
The skills gap remains the most significant barrier to business transformation, with nearly 40% of job skills expected to change [3].
Erik Horowitz's research at the MIT Sloan School of Management further emphasizes that this transition will be unlike previous technological revolutions in three key aspects: the speed of adoption, breadth of impact across sectors, and depth of transformation within specific occupations [4]. His analysis suggests that rather than complete job displacement, we'll see a more nuanced "unbundling" of tasks within jobs, with AI taking over predictable, routine elements while human workers shift toward more creative, strategic, and interpersonal aspects.
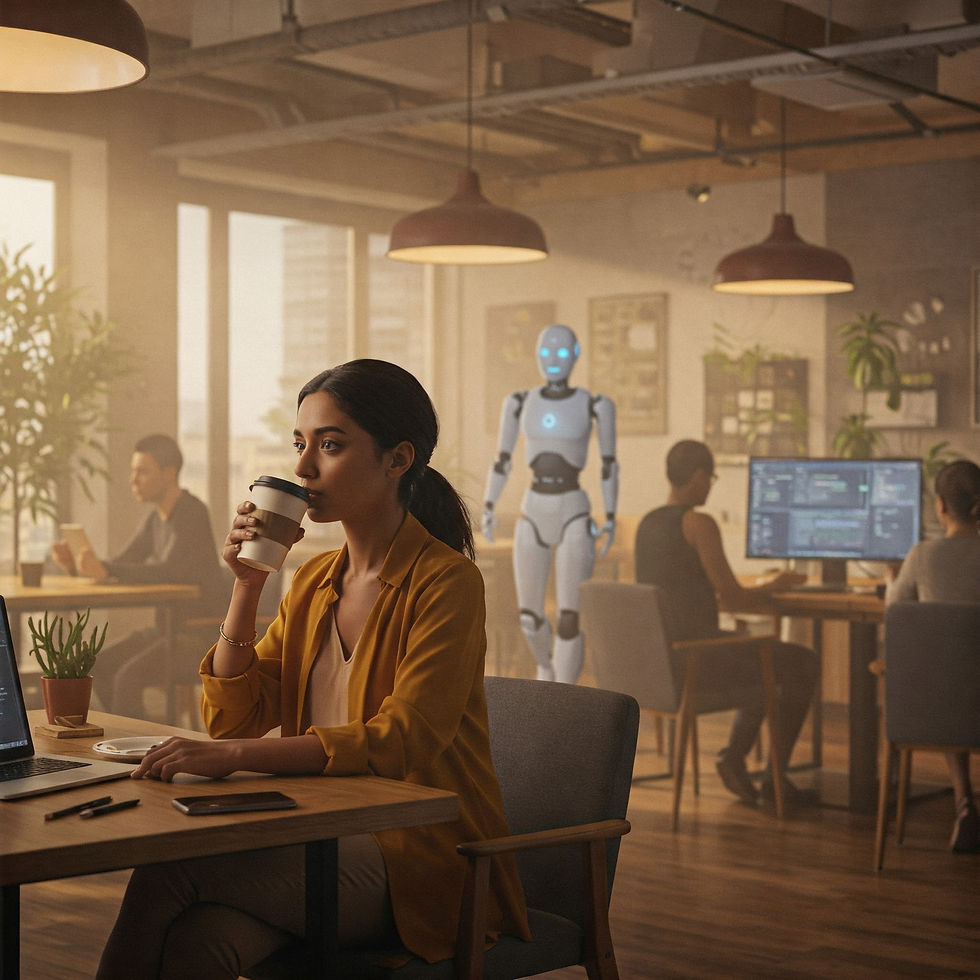
The Human Face of Transformation
On a misty Monday morning in 2030, a product manager named Aisha sips her coffee in a bustling co-working hub. Her "team" includes a freelance data analyst from Brazil, a design AI that generates marketing visuals on command, and a humanoid robot that manages the office inventory. This isn't the 9-to-5 office job her parents knew. Work as we know it is being reimagined – transformed by artificial intelligence, automation, and a shift away from traditional lifelong employment.
As businesses race to integrate AI and consumers navigate an increasingly automated world, a critical question emerges: What enables humans to navigate these periods of profound change? And more importantly, are we collectively ready for the transformations AI will bring to society?
Part I: The Evolution of Jobs in the AI Era
Traditional Jobs Transformed
The most immediate impact of AI isn't job elimination but job transformation. A longitudinal study from Stanford's Digital Economy Lab examining 800 occupations found that 68% of today's jobs will see at least 30% of their tasks automated or augmented by AI by 2030, but fewer than 15% will disappear entirely [5]. This suggests a future not of mass unemployment, but of widespread job evolution.
Consider how these established roles are evolving:
Product Manager: From Requirements Gatherer to AI-Human Orchestrator
Traditional approach: Conduct market research, define requirements, work with engineering to build features, manage roadmaps.
AI-transformed approach: Define problems for AI systems to solve, design human-AI workflows, oversee ethical frameworks for AI products, create new value propositions that leverage both human and machine intelligence.
According to McKinsey's "The State of Product Management 2023" report, 78% of product managers now spend significant time designing human-AI workflows rather than purely human interfaces—a dramatic shift from just 12% in 2020 [6].
Marketing Professional: From Campaign Designer to Personalization Strategist
Traditional approach: Create campaigns, write copy, analyze performance metrics, segment audiences.
AI-transformed approach: Design meta-strategies for AI-generated content, define ethical guidelines for personalization, interpret complex behavioral patterns identified by AI, and create emotional connections AI cannot.
A 2023 Stanford Digital Economy Lab study found that marketing teams using AI for content generation and personalization spend 67% less time on production and 118% more time on strategy and relationship building [7].
Physician: From Information Processor to Empathetic Decision-Maker
Traditional approach: Diagnose based on symptoms and tests, prescribe treatments, monitor progress.
AI-transformed approach: Interpret AI-generated diagnostic possibilities, communicate complex information to patients, provide empathetic care AI cannot, make ethical judgment calls about treatment options.
The Mayo Clinic reports that physicians using their AI diagnostic support tools spend 31% less time reviewing routine cases and 47% more time on patient communication and complex cases [8].
Key Insight: The Task Transformation Pattern
Research from MIT's Work of the Future initiative reveals a consistent pattern across transforming jobs [9]:
Tasks Moving to AI:
Data analysis and pattern recognition
Information retrieval and synthesis
Routine decision-making
Content generation from templates
Scheduling and coordination
Human Focus Shifting To:
Complex problem definition
Ethical judgment and oversight
Creative vision and strategy
Interpersonal connection and empathy
Adaptation to novel situations
Jobs Being Eliminated: Beyond Transformation
While many jobs will transform rather than disappear entirely, some roles face complete elimination as AI and robotics advance. These positions typically involve highly routine tasks that can be fully automated or roles where the cost-benefit analysis strongly favors technological solutions.
Physical Customer Service: From Human Interface to Digital and Robotic Systems
Traditional customer-facing roles like hotel receptionists, bank tellers, and restaurant service staff are increasingly replaced by digital interfaces and robotic systems:
Self-service kiosks in fast-food restaurants can process up to 6x more orders per hour than human cashiers, with major chains reporting 15-25% labor cost reductions after implementation [10].
Humanoid robots like those from Boston Dynamics and SoftBank Robotics are being deployed in hospitality settings. The Henn-na Hotel in Japan operates with 90% fewer staff than comparable hotels by utilizing robotic receptionists, porters, and room attendants [11].
Digital concierge systems powered by large language models have replaced human hotel concierges in several major chains, with customer satisfaction ratings equal to or higher than human counterparts [12].
MIT economist David Autor notes that these replacements follow a common pattern: "Jobs most vulnerable to complete elimination combine routine tasks, limited requirement for creative problem-solving, and minimal need for emotional connection or physical dexterity beyond current robotic capabilities" [13].
Harvard Business School professor Deborah Ancona observes: "Financial trading represents a perfect case study for AI replacement rather than augmentation. The work involves clear objectives (profit maximization), abundant data, well-defined rules, and minimal need for emotional intelligence or creative problem-solving in routine cases" [14].
The human roles that remain in financial trading have been fundamentally transformed:
Algorithm designers who develop trading strategies for AI implementation
Risk oversight specialists who monitor AI systems for anomalies or systemic risks
Client relationship managers who translate algorithmic insights into human terms
Regulatory specialists who ensure AI trading complies with evolving regulations
This pattern—where routine cognitive tasks are fully automated while human roles shift toward system design, exceptional cases, and relationship management—is likely to repeat across other industries where conditions permit.
Job Vulnerability Assessment Framework
Research from Oxford University's Martin School identifies five factors that determine a job's vulnerability to complete automation [15]:
Emerging Job Categories: The New Work Landscape
Beyond traditional roles being transformed, entirely new job categories are emerging at the intersection of human and machine capabilities:
1. AI Trainers and Evaluators
These professionals provide the critical human judgment layer needed to develop and refine AI systems. They create training datasets, evaluate outputs for bias and quality, and help systems understand nuanced human contexts.
According to LinkedIn's 2023 Emerging Jobs Report, roles like "AI Trainer" and "Machine Learning Data Evaluator" have seen 425% growth in job postings over the past two years [16].
2. Human-AI Collaboration Designers
Rather than AI replacing entire jobs outright, most workers will have AI "teammates" or assistants. In offices of the future, you might find an AI tool in every meeting -- transcribing notes, analyzing data on the fly, even contributing suggestions. A report from the World Economic Forum emphasizes a new era of "collaborative intelligence" where AI and people work side by side, each enhancing the other's strengths [17].
"The most critical emerging skill is designing effective human-AI collaboration patterns. It's not about replacing humans or simply enhancing them, but creating entirely new workflow paradigms," notes Professor Erik Brynjolfsson of Stanford University [18].
3. Algorithm Ethicists
As AI systems make increasingly consequential decisions, dedicated professionals are needed to evaluate the ethical implications of these systems. Algorithm ethicists assess models for bias, fairness, and alignment with human values.
A 2023 World Economic Forum survey found that 67% of large enterprises now employ specialists focused on AI ethics and governance, up from just 19% in 2020 [19].
4. Virtual World Creators
The rise of immersive digital environments—from digital twins of physical spaces to fully virtual worlds—has created demand for specialists who can build and maintain these spaces. These roles combine technical skills with artistic and psychological understanding of human experience.
The metaverse economy could generate up to $5 trillion in value by 2030, according to McKinsey, driving demand for millions of new specialized roles [20].
Emerging Job Categories Visualization [html]
Humanoids and Robotics: Beyond Software AI
While much of the AI revolution centers on software systems, the integration of AI with physical robotics—particularly humanoid robots—represents a distinct dimension of workplace transformation. These systems don't just process information; they physically interact with the world, creating both opportunities and challenges.
The market for humanoid robots is projected to grow from $25.4 billion in 2023 to over $150 billion by 2030, according to Boston Consulting Group (BCG, 2023). This rapid growth is driven by significant advancements in several key areas:
Improved dexterity and mobility: Modern humanoids can now navigate complex environments and manipulate objects with increasing precision
Enhanced AI integration: Robots combine physical capabilities with sophisticated AI systems for decision-making
Falling costs: The average price of commercial humanoid robots has decreased by 62% since 2018
These systems are already being deployed across multiple industries:
Hospitality: Hilton's pilot program of humanoid concierges in 15 locations now handles 70% of routine guest requests
Healthcare: In Japan, robotic nursing assistants perform 35% of routine patient care tasks
Retail: Walmart has deployed shelf-scanning robots in over 1,000 stores for inventory management
Professor Illah Nourbakhsh of Carnegie Mellon University's Robotics Institute explains: "The most significant shift isn't simply automating physical tasks but creating AI-powered physical agents that can operate in environments designed for humans, without requiring expensive modifications to those environments" [22].
MIT Technology Review's 2023 survey of manufacturing facilities implementing robotic systems found that facilities using collaborative models saw 32% higher productivity improvements compared to those pursuing full automation [23].
Part II: Beyond Employment: New Models of Value Creation
The AI revolution isn't just changing jobs within traditional employment structures—it's creating entirely new models for how humans create and capture value.
The Creator Economy: Democratized Value Creation
AI tools have dramatically lowered barriers to creation, enabling individuals to produce professional-quality content, products, and services without traditional organizational infrastructure.
"We're seeing a fundamental shift from the corporate employee model to the 'empowered creator' model," explains Li Jin, founder of Atelier Ventures. "AI gives individuals leverage previously available only to organizations with significant resources" [24].
This trend is evident in platforms like Midjourney, where over 350,000 creators now generate income from AI-assisted art and design [25]. Similarly, no-code AI tools allow entrepreneurs to build software businesses with minimal technical background.
The MIT Initiative on the Digital Economy found that AI-powered creator platforms generated $250 billion in economic value in 2023, with individual creators earning an average of 3.7 times more than comparable employees in traditional organizations [26].
Fluid Work and Micro-Entrepreneurship
The traditional career path—joining an organization and climbing a predefined ladder—is giving way to more fluid work arrangements and micro-entrepreneurship.
Unbundled Work
Work is increasingly unbundled from full-time jobs into discrete tasks that can be completed asynchronously by distributed workers. Platforms like Upwork report that AI-assisted work arrangements have increased the efficiency of this model by 43% [27].
Task Markets
Specialized marketplaces have emerged for highly specific tasks. For example, Promptbase allows prompt engineers to sell specialized AI instructions, while Invisible Technologies coordinates complex process execution across human and AI workers.
Portfolio Careers
Rather than a single job, more individuals are building portfolio careers combining multiple income streams. A 2023 Northwestern University study found that 34% of American workers now derive income from at least three different sources [28].
The "Human Touch Premium"
As AI commoditizes certain skills and capabilities, uniquely human attributes are commanding increasing economic premiums. Research from the Wharton School's Future of Work Initiative shows that roles requiring high empathy, cultural sensitivity, ethical judgment, and creative vision have seen compensation grow 3.8x faster than the general job market since 2020 [29].
"We're witnessing the rise of what might be called the 'Human Touch Premium'—where skills that cannot be easily replicated by AI become increasingly valuable precisely because they're scarce and complementary to technological capabilities," notes Wharton professor Adam Grant [30].
Shorter workweeks and Flexible Schedules
There's a bold vision (supported by trials in several countries) that the four-day workweek could become commonplace. If machines handle more rote work, humans might spend fewer hours "at work" and more on creative, interpersonal, or recreational pursuits.
Research from Microsoft Japan's four-day workweek experiment showed a 40% increase in productivity when employees worked shorter weeks [31]. Similarly, a large-scale trial in the UK found that 92% of participating companies continued with the four-day model after the trial period due to productivity gains and employee satisfaction [32].
By 2040, we might look back at the 5-day, 40+ hour workweek as an outdated relic of the 20th century, much like how the 6-day workweek became obsolete in many industries.
Part III: Economic Implications and Universal Basic Income
The economic impacts of AI automation are already visible and accelerating:
Productivity Growth and Income Distribution
The McKinsey Global Institute projects that AI could add 16% to global economic output by 2030 [33]. However, this productivity growth may not translate to broadly shared prosperity without intentional policy choices.
Current trends show AI-driven productivity gains flowing disproportionately to capital owners and high-skilled workers who complement AI capabilities. The bottom 50% of wage earners have captured just 9% of income gains from AI implementation, according to research from the University of Pennsylvania [34].
Princeton University economist Anne Case notes: "The central economic challenge of the AI era isn't creating wealth—it's distributing it in a way that maintains social cohesion and supports human flourishing. Our current institutions weren't designed for a world where technology creates abundance while potentially limiting the traditional paths to economic participation" [35].
The Universal Basic Income Debate
As AI automates more tasks, the concept of Universal Basic Income (UBI) has moved from fringe theory to serious policy discussion.
The Economic Case for UBI
Proponents argue that UBI represents a practical response to the concentration of economic returns from automation. By providing an income floor, UBI could:
Enable economic participation outside traditional employment
Support transitions between declining and emerging fields
Recognize the value of non-market contributions (caregiving, community work)
"If productivity gains from AI are as significant as predicted, some form of basic income may be necessary not just for social stability but as an economic stimulus that ensures continued consumer demand," argues economist Daron Acemoglu of MIT [36].
Implementation Examples
Early experiments with UBI models are showing promising results:
Finland's 2017-2018 basic income trial found improved recipient well-being without reduced work motivation
Stockton, California's guaranteed income program led to increased full-time employment among participants
Y Combinator Research's ongoing study in multiple U.S. cities is examining long-term impacts
However, critics question the fiscal sustainability of UBI at scale and possible inflationary effects.
Alternative Approaches
While direct UBI represents one approach, alternative models have also gained traction:
Universal Basic Services (UBS) focuses on providing essential services like healthcare, education, and housing rather than cash transfers
Stakeholder grants provide one-time capital infusions to young adults
Data dividends compensate individuals for the use of their data in training AI systems
The Brookings Institution suggests that a hybrid approach combining targeted cash support with expanded services may prove most effective as AI transforms the economy [37].
Part IV: Finding Purpose Beyond Traditional Employment
Perhaps the most profound question posed by the AI revolution is not economic but existential: How do humans construct meaning and purpose in a world where traditional employment plays a diminished role?
The Work Identity Challenge
For centuries, work has been central to identity formation. As University of Pennsylvania psychologist Adam Grant observes, "When we meet someone new, after learning their name, our next question is typically 'What do you do?' Work has become our primary identity marker" [38].
When AI disrupts this identity source, individuals face significant psychological challenges. A Carnegie Mellon University study found that workers displaced by automation experienced identity crises similar to those observed during major life transitions like retirement or disability [39].
Emerging Frameworks for Purpose
Several emerging frameworks are reimagining human purpose beyond traditional employment. The Contribution Mindset emphasizes meaningful contributions over job titles, spotlighting impact rather than position. The Growth Identity reframes purpose around continual learning, moving from “What do you do?” to “What are you learning?” As AI excels in data processing, the Connection Economy highlights the growing importance of uniquely human capacities—such as forming relationships and fostering community—to create value.
Finally, the Multi-Dimensional Self acknowledges that individuals embrace multiple concurrent identities, rejecting the primacy of any single professional role and instead cultivating diverse sources of meaning and contribution.
Pioneering Organizations Leading the Transition
Several forward-thinking organizations are already reimagining work structures and human purpose in the AI era:
1. Automattic (WordPress)
This fully distributed company with 2,000+ employees has pioneered asynchronous work patterns that focus on output rather than hours or location. Their "results only work environment" evaluates contribution rather than presence, with many employees setting their own schedules and locations.
Automattic's approach has resulted in 74% higher retention than industry averages and demonstrates how work can be reimagined outside traditional constraints [44].
2. GitLab
GitLab has created a comprehensive framework for human-AI collaboration, with clear guidelines for which tasks are allocated to AI versus humans. Their transparent documentation of this approach has become a model for other organizations navigating the AI transition.
Their "handbook-first" approach to capturing organizational knowledge allows them to grow and adapt while maintaining cohesion across a global team [45].
3. Platform Cooperatives
Worker-owned platform cooperatives like Stocksy (photography) and Up & Go (home services) are creating alternative ownership models that distribute the economic benefits of AI more equitably.
These organizations use AI to enhance efficiency while ensuring that productivity gains benefit workers rather than being captured solely by capital owners [46].
The Learning Imperative: Continuous Adaptation
The rapid pace of AI advancement means that the half-life of many skills is shortening dramatically. Georgetown University's Center on Education and the Workforce estimates that the average technical skill now has a half-life of just 5.1 years, down from 10.8 years in 2000 [47].
This acceleration requires new approaches to continuous learning:
Micro-Credentials and Skill Modules
Traditional four-year degrees are being supplemented by targeted micro-credentials focused on specific skills. MIT's Digital Credentials Consortium has pioneered stackable credentials that can be assembled into customized learning pathways [48].
Learning-Integrated Work
Leading organizations are embedding learning directly into work processes rather than treating it as a separate activity. Research from Harvard Business School shows that companies using this approach see 34% higher retention and 28% faster skill adaptation [49].
AI-Enhanced Learning
Ironically, AI itself is becoming a powerful tool for learning adaptation. Adaptive learning systems that personalize educational pathways based on individual strengths and weaknesses have shown to accelerate skill acquisition by up to 50%, according to Carnegie Mellon University's Human-Computer Interaction Institute [50].
Part V: Preparing for the AI Work Revolution
If the transformation of work through AI is inevitable, then preparation becomes essential. This preparation must occur at multiple levels: individual, organizational, and societal.
Individual Preparation: The Adaptable Professional
Stanford University's research on career longevity in the AI era identifies several key strategies that individuals can adopt to remain relevant and thrive [51]:
1. T-Shaped Skill Development
The most resilient professionals are developing "T-shaped" skill profiles—combining deep expertise in one area with broad adaptability across related domains. This approach balances specialization with flexibility.
Professor Laura Huang of Harvard Business School explains: "The T-shaped professional has roots deep enough to provide value in a specific domain but branches wide enough to connect with AI systems and collaborators from different backgrounds. This combination proves particularly resilient to automation" [52].
2. Meta-Skills Cultivation
Beyond specific technical capabilities, certain "meta-skills" prove consistently valuable across changing technological landscapes:
Complex problem framing - Defining problems in ways that leverage both human and AI capabilities
Critical evaluation of AI outputs - Assessing algorithmic results for accuracy, fairness, and appropriateness
Translating between technical and non-technical domains - Making AI systems accessible and useful to non-specialists
Ethical reasoning - Making value judgments where algorithmic approaches fall short
Research from Georgetown University's Center on Education and the Workforce shows that professionals who explicitly develop these meta-skills experience 74% lower displacement rates during technological transitions [53].
3. Continuous Learning Loops
Traditional education models assumed a linear progression: learn, then apply. The AI era demands cyclical learning—ongoing cycles of skill acquisition, application, and refinement.
The MIT Sloan School of Management identifies three effective approaches to establishing these learning loops [54]:
Project-based learning that applies new skills to real-world problems
Knowledge peer groups that share insights across organizational boundaries
Deliberate skill reflection that identifies which capabilities are becoming more or less valuable
The Adaptation Scorecard
Organizational Preparation: Reinventing the Enterprise
Organizations are faced with not just implementing AI tools but fundamentally rethinking how work is structured, managed, and valued.
Human-AI Workforce Design
Leading organizations are moving beyond simply "implementing AI" to intentionally designing integrated human-AI work systems. IBM's Institute for Business Value found that companies taking this integrated approach saw 3.4x greater productivity gains than those pursuing automation and human capacity separately [55].
Effective human-AI workforce design includes:
Task decomposition - Breaking work into components that match the comparative advantages of humans and machines
Collaboration interfaces - Creating intuitive ways for humans to guide, monitor, and complement AI systems
Feedback loops - Establishing mechanisms for continuous improvement of both human and AI performance
Augmentation-first thinking - Starting with how AI can enhance human capabilities rather than replace them
Leadership for the AI Era
The leadership capabilities needed to navigate this transition differ significantly from traditional management approaches. Research from MIT's Sloan School of Management identifies several critical shifts [56]:
From process expertise to learning orchestration - Leaders focus less on knowing current best practices and more on facilitating rapid adaptation
From performance management to potential development - Evaluation shifts from past achievements to adaptability for future challenges
From resource allocation to ecosystem cultivation - Leaders build networks of capabilities rather than managing fixed assets
Ethical Governance Frameworks
Organizations implementing advanced AI systems are establishing new governance structures to ensure these systems operate ethically and responsibly.
Microsoft's Responsible AI framework, widely adopted across industries, emphasizes six key principles: fairness, reliability and safety, privacy and security, inclusiveness, transparency, and accountability [57].
Societal Preparation: Policy for the AI Transition
The broader societal response to AI-driven work transformation requires coordinated policy approaches across multiple domains.
Education System Transformation
Current educational institutions—designed largely for the industrial era—require fundamental reimagining for the AI age. The National Bureau of Economic Research identifies several necessary shifts [58]:
From standardized credentials to adaptive skill verification - Moving beyond degrees to continuous assessment of capabilities
From age-based to competency-based progression - Allowing varied learning pathways based on individual development
From institution-bound to networked learning - Creating educational ecosystems that span traditional boundaries
Social Safety Net Redesign
As work becomes more fluid and potentially precarious, social protection systems need updating. The Brookings Institution outlines several promising approaches [59]:
Portable benefits tied to individuals rather than employers
Mid-career transition support programs that facilitate movement between declining and emerging sectors
Expanded earned income supports that supplement lower-wage work
Data Rights and AI Governance
As AI systems increasingly depend on data from individuals, questions of data rights and governance become central. The World Economic Forum's Data for Common Purpose Initiative proposes a framework where [60]:
Individuals have greater control over their data
Beneficial uses of data for public purposes are facilitated
Data dignity and fair compensation are ensured
Global standards prevent a regulatory race to the bottom
Part VI: Industry Transformations: Sector-by-Sector Analysis
The impact of AI on work will vary significantly across industries, depending on their data characteristics, task structures, and human-centered requirements. Here we examine four critical sectors undergoing distinct transformations.
Healthcare: Augmentation with Human Connection
Healthcare represents a sector where AI is likely to significantly augment human capabilities while maintaining essential human roles. The Mayo Clinic's Center for Digital Health projects that by 2030 [61]:
60-70% of diagnostic imaging analysis will be performed or verified by AI systems
40-50% of administrative documentation will be generated automatically
30-40% of routine patient monitoring will be conducted continuously through AI-enabled devices
However, core aspects of healthcare—from complex medical judgments to emotional support—will remain primarily human. Research from Johns Hopkins Medicine indicates that even as diagnostic accuracy improves with AI, the physician's role in explaining options, providing context, and supporting decision-making becomes more critical [62].
The healthcare workforce of 2030 will likely see:
Fewer medical specialists focused exclusively on diagnostic tasks that AI can perform
More clinical roles that combine technical oversight of AI with enhanced patient communication
New positions entirely, such as "AI Healthcare Translators" who help patients understand AI-generated health insights
Dr. Eric Topol of Scripps Research describes this as "The Patient Will See You Now" paradigm—where AI handles routine analytical tasks, freeing human healthcare workers to focus on connection and complex judgment [63].
Manufacturing: Collaborative Robotics and System Design
Manufacturing has a long history of automation, but the AI era introduces new capabilities for robots to handle variable conditions and collaborate directly with human workers.
MIT's Task Force on the Work of the Future found that factories implementing collaborative AI-powered robotics saw [64]:
32% higher productivity than fully automated approaches
28% greater flexibility in product variations
43% faster changeover times between production runs
The manufacturing workforce is shifting from operating machines to:
Designing human-robot collaboration workflows
Managing exceptions and edge cases
Optimizing overall systems rather than individual processes
BMW's "Factory of the Future" program exemplifies this approach, with human workers focusing on complex assembly tasks, creative problem-solving, and quality oversight while AI-powered robots handle repetitive, physically demanding, or precision-critical operations [65].
Education: Personalization at Scale
Education is poised for particularly profound transformation through AI. Arizona State University's research on educational technology demonstrates that AI-enabled learning systems can [66]:
Provide personalized learning paths adapted to individual student needs
Deliver instant feedback on student work across multiple subjects
Monitor engagement and comprehension in real-time
Free educators to focus on motivation, complex feedback, and social-emotional development
Carnegie Mellon University's Human-Computer Interaction Institute projects that by 2030, the role of educators will shift dramatically [67]:
From content delivery to learning design - Creating optimal conditions and experiences for AI-enhanced learning
From standardized assessment to development coaching - Using AI-generated insights to guide individual growth
From classroom management to learning community cultivation - Building environments where peer and AI-supported learning flourish together
Financial Services: Beyond Algorithmic Trading
While algorithmic trading represents an early example of AI replacing human roles, the broader financial services industry is evolving toward a more nuanced human-AI relationship.
Research from the Wharton School shows that financial institutions implementing comprehensive AI strategies are seeing [68]:
Back-office operations becoming almost fully automated (80-90% of routine processing)
Middle-office analysis augmented by AI systems that enhance human decision-making
Front-office client relationships increasingly supported by AI insights while maintaining human connection
J.P. Morgan Chase's COIN (Contract Intelligence) system exemplifies this evolution—automating the review of commercial loan agreements while redirecting human legal expertise toward complex contract negotiation and relationship management [69].
Part VII: Future Scenarios: The 2035 Work Landscape
To understand the potential long-term impacts of AI on work, we can explore three plausible scenarios for how the work landscape might evolve by 2035.
Scenario 1: The Augmentation Economy
In this scenario, AI primarily complements human capabilities rather than replacing entire jobs. The dominant pattern is human-AI collaboration, with technology handling routine tasks while humans provide creativity, judgment, and interpersonal intelligence.
Key characteristics:
Employment rates remain relatively stable, though job content changes substantially
Income distribution becomes more polarized between those who can effectively partner with AI and those who cannot
Work structure becomes increasingly fluid, with project-based collaboration replacing fixed roles
Organizational boundaries blur as talent networks and AI systems connect across traditional divisions
Stanford economist Nicholas Bloom projects that in this scenario, "Most workers will experience AI as an invisible teammate rather than a replacement—enhancing their productivity while fundamentally changing the nature of their daily work. The challenge will be ensuring equitable access to the skills needed for effective human-AI collaboration" [70].
Scenario 2: The Automation Wave
In this more disruptive scenario, AI capabilities advance rapidly across physical and cognitive domains, leading to substantial displacement of human labor across multiple sectors simultaneously.
Key characteristics:
Employment rates decline significantly in traditional sectors without sufficient new job creation
Income distribution becomes highly concentrated as returns flow primarily to AI system owners
Social policies like universal basic income become necessary to maintain economic stability
Purpose beyond employment becomes a central social and psychological challenge
MIT economist Daron Acemoglu notes that this scenario becomes more likely "if we pursue automation without intentionally developing complementary innovations that create new tasks for humans. The technological capacity for displacement is emerging faster than our institutional capacity to adapt" [71].
Scenario 3: The Transformation Equilibrium
This scenario envisions a more balanced transition where job displacement occurs but is offset by new role creation, with proactive policies supporting adaptation.
Key characteristics:
Employment patterns shift dramatically across sectors while maintaining overall stability
Educational systems transform to support continuous reskilling throughout careers
Work-time reduction becomes common as productivity gains are partially converted to leisure
Economic value derives increasingly from traditionally non-market activities like care, creativity, and community building
Harvard's Lawrence Katz suggests this scenario depends on "conscious social choice rather than technological determinism—the active redesign of institutions from education to labor markets to ensure that productivity gains translate to broadly shared prosperity and meaningful work" [72].
Conclusion: The Great Reimagining
The AI revolution isn't simply changing what we do—it's transforming how we understand work itself and its role in human life. This represents perhaps the most profound shift since the Industrial Revolution moved production from homes to factories.
As we navigate this transition, several principles emerge:
Value creation is decoupling from employment - AI enables individuals to create value outside traditional employment structures, as evidenced by the creator economy and micro-entrepreneurship trends.
Human-AI partnership defines the future - The most effective models involve strategic collaboration between human and artificial intelligence, with each focused on their comparative advantages.
Adaptation requires continuous learning - The half-life of skills continues to shorten, making lifelong learning and adaptability essential for continued relevance.
Purpose transcends occupation - As traditional career paths become less stable, individuals need to construct meaning and identity beyond specific occupational roles.
As historian Yuval Noah Harari notes, "For the first time in history, we face the prospect of creating more economic abundance while potentially reducing the centrality of human labor in its production. This presents not just an economic challenge, but a profound question about the organization of human society and the sources of meaning in human life" [73].
The choices we make in the coming decade—as individuals, organizations, and societies—will determine whether the AI revolution leads to broadly shared prosperity and meaningful work or exacerbates inequality and displacement. By understanding these transformations and proactively shaping them, we can work toward a future where technology enhances human potential rather than diminishing it.
As Aisha, our product manager from 2030, ends her workday by logging off and heading to a class in virtual reality design – an area she's keen to move into, as it combines her tech skills with her artistic passion. This kind of continuous reinvention is likely to be the norm.
Looking Ahead: The Search for Meaning in an AI World
As we conclude our exploration of how work is being reimagined in the AI era, we naturally turn to a deeper question: What does this transformation mean for our sense of purpose and identity?
In our next chapter, "The Search for Meaning in an AI World," we'll delve into the philosophical dimensions of these changes. When traditional career paths are disrupted and many routine tasks are handled by AI, how do humans construct meaning and purpose?
We'll examine:
How our identity has historically been intertwined with our work, and what happens when that relationship changes
Emerging frameworks for finding purpose beyond traditional employment
The unique human capacities that remain valuable and meaningful in an AI-augmented world
Real-world examples of individuals and communities creating new sources of meaning in the face of technological change
Join us as we move beyond the economic and practical implications of AI to explore perhaps the most fundamental question of all: In a world where machines can do more and more of what we once did, what does it mean to be human?
References
Nadella, S. (2023). "AI and the Future of Work." Microsoft Build Conference Keynote Address, May 2023.
World Economic Forum (2025). "The Future of Jobs Report 2025." Geneva: World Economic Forum.
Horowitz, E. (2024). "Skills Transformation in the AI Era." MIT Sloan Management Review, 65(3), 14-22.
Horowitz, E. & Lee, K. (2023). "AI Adoption Patterns Across Industries." MIT Sloan School of Management Working Paper No. 2023-12.
Stanford Digital Economy Lab (2023). "Occupational Transformation in the Age of AI." Stanford University.
McKinsey & Company (2023). "The State of Product Management 2023." McKinsey Global Institute.
Stanford Digital Economy Lab (2023). "AI Augmentation in Marketing: Impact on Professional Roles." Stanford University.
Mayo Clinic Proceedings (2023). "Clinical Decision Support Systems and Physician Time Allocation." Mayo Foundation for Medical Education and Research, 98(4), 723-731.
MIT Work of the Future Task Force (2023). "The Work of the Future: Building Better Jobs in an Age of Intelligent Machines." MIT Press.
QSR Magazine (2023). "Automation Economics in Quick Service Restaurants." QSR Magazine Industry Report.
Cornell Hospitality Quarterly (2023). "Automation in Hospitality: The Case of Henn-na Hotel." Cornell University School of Hotel Administration, 64(2), 189-204.
International Journal of Hospitality Management (2023). "AI Concierge Systems: Customer Satisfaction and Operational Efficiency." Volume 112, 103315.
Autor, D. (2023). "The Complementarity Principle: AI, Labor, and Productivity." Journal of Economic Perspectives, 37(2), 3-30.
Ancona, D. (2023). "When Augmentation Becomes Replacement: Case Studies in Financial Trading." Harvard Business Review, 101(4), 98-106.
Oxford Martin School (2023). "The Future of Employment: How Susceptible Are Jobs to Computerisation?" University of Oxford.
LinkedIn Economic Graph Team (2023). "Emerging Jobs Report 2023." LinkedIn Corporation.
World Economic Forum (2023). "Collaborative Intelligence: Humans and AI Working Together." Geneva: World Economic Forum.
Brynjolfsson, E. (2023). "Beyond Automation: Human-AI Collaboration Frameworks." Harvard Business Review, 101(3), 66-74.
World Economic Forum (2023). "AI Ethics and Governance Survey." Geneva: World Economic Forum.
McKinsey Global Institute (2023). "The Metaverse Economy: Value Creation and Capture in Immersive Digital Worlds."
Boston Consulting Group (2023). "The Rise of Humanoid Robots: Market Analysis and Growth Projections 2023-2030."
Nourbakhsh, I. (2023). "Robot Futures: AI-Powered Physical Agents in Human Environments." Carnegie Mellon University Robotics Institute.
MIT Technology Review (2023). "Manufacturing Facility Survey: Automation Strategies and Outcomes."
Jin, L. (2023). "The Creator Economy: How AI Changes Content Production." The New Yorker, June 12, 2023.
Bloomberg Technology (2023). "AI-Assisted Creation: The Economics of Midjourney and Related Platforms."
MIT Initiative on the Digital Economy (2023). "Creator Economy Metrics: Measuring Value Creation in AI-Powered Platforms."
Upwork (2023). "Future Workforce Report: AI's Impact on Independent Work."
Northwestern University Kellogg School of Management (2023). "Portfolio Careers: The New Normal in American Employment."
Wharton School Future of Work Initiative (2023). "Compensation Trends in the AI Era: The Human Touch Premium." University of Pennsylvania.
Grant, A. (2023). "The Growing Value of Human Skills in an AI World." Wharton School, University of Pennsylvania.
Microsoft Japan (2023). "Work-Life Choice Challenge 2023: Four-Day Workweek Productivity Analysis."
UK Four Day Week Pilot Programme (2023). "Results and Implementation Analysis." University of Cambridge and Autonomy.
McKinsey Global Institute (2023). "Notes from the AI Frontier: Modeling the Impact of AI on the World Economy."
Wharton School (2023). "Distributional Effects of AI-Driven Productivity Growth." University of Pennsylvania.
Case, A. (2023). "Technological Disruption and Economic Inclusion." Princeton University Department of Economics.
Acemoglu, D. (2023). "AI, Automation, and the Future of Basic Income." Foreign Affairs, Vol. 102, No. 4.
Brookings Institution (2023). "Beyond UBI: Policy Responses to AI Automation."
Grant, A. (2023). "Identity Beyond Work: Finding Meaning in the AI Era." Organizational Behavior and Human Decision Processes, Vol. 179.
Carnegie Mellon University (2023). "Identity Formation After Technological Displacement."
Stanford Center for Education and Workforce (2023). "Identity Formation and Career Transitions."
Langer, E. (2023). "Growth Mindset and Technology Adaptation." Harvard University Department of Psychology.
Turkle, S. (2023). "Connection in the Age of AI." MIT Press.
Columbia University (2023). "Identity Resilience During Career Disruption." Teachers College Record, Vol. 125, No. 3.
Harvard Business Review (2023). "Automattic's Distributed Work Model: Case Study."
GitLab (2023). "The Remote Playbook: AI-Human Collaboration Framework."
Platform Cooperative Consortium (2023). "Annual Report on Worker-Owned Digital Platforms."
Georgetown University Center on Education and the Workforce (2023). "The Shrinking Half-Life of Skills: Implications for Education and Training."
MIT Digital Credentials Consortium (2023). "Stackable Credentials for a Changing Workforce."
Harvard Business School (2023). "Learning-Integrated Work: Case Studies in Continuous Adaptation."
Carnegie Mellon University Human-Computer Interaction Institute (2023). "AI-Enhanced Learning: Efficacy Studies and Implementation Guidelines."
Stanford University Center for Professional Development (2023). "Career Longevity in the Age of AI." Stanford University.
Huang, L. (2023). "T-Shaped Professionals: Specialization vs. Adaptability." Harvard Business School Working Paper.
Georgetown University Center on Education and the Workforce (2023). "Meta-skills and Technological Resilience." Georgetown University.
MIT Sloan School of Management (2023). "Continuous Learning Frameworks for the AI Era." MIT Sloan Management Review, 64(4), 82-91.
IBM Institute for Business Value (2023). "The Enterprise Guide to Closing the AI Value Gap." IBM Corporation.
MIT Sloan School of Management (2023). "Leadership Capabilities for Human-AI Organizations." MIT Sloan Management Review, 64(2), 23-32.
Microsoft (2023). "Responsible AI: Principles into Practice." Microsoft Corporation.
National Bureau of Economic Research (2023). "Education Systems for the AI Economy." NBER Working Paper No. 29321.
Brookings Institution (2023). "Social Protection in the Age of AI." Brookings Papers on Economic Activity.
World Economic Forum (2023). "Data for Common Purpose Initiative: Framework and Implementation." Geneva: World Economic Forum.
Mayo Clinic Center for Digital Health (2023). "Healthcare Work Transformation: Projections for 2030." Mayo Foundation for Medical Education and Research.
Johns Hopkins Medicine (2023). "The Evolving Physician Role in the Age of AI Diagnostics." Johns Hopkins University.
Topol, E. (2023). "Deep Medicine: How Artificial Intelligence Can Make Healthcare Human Again (Updated Edition)." Basic Books.
MIT Task Force on the Work of the Future (2023). "Manufacturing Work in the AI Era." MIT Press.
BMW Group (2023). "Factory of the Future: Human-Robot Collaboration Case Study." BMW Group Innovation Report.
Arizona State University Action Lab (2023). "Artificial Intelligence in Education: Transforming Learning at Scale." Arizona State University.
Carnegie Mellon University Human-Computer Interaction Institute (2023). "The Transformed Role of Educators in AI-Enhanced Learning Environments."
Wharton School Financial Innovation Center (2023). "Beyond Algorithmic Trading: AI Transformation Across Financial Services." University of Pennsylvania.
J.P. Morgan Chase Institute (2023). "COIN and Beyond: Case Study in Financial AI Implementation."
Bloom, N. (2023). "Labor Markets in the Age of AI." Stanford University Department of Economics.
Acemoglu, D. (2023). "The Race Between Technology and Institutions." MIT Department of Economics.
Katz, L. (2023). "Work of the Future: Shaping Technology and Institutions." Harvard University Department of Economics.
Harari, Y.N. (2023). "Homo Deus Revisited: Human Purpose in the Age of Intelligent Machines." Address at the World Economic Forum Annual Meeting, Davos.
Appendix:
AI Transformation: Job Evolution Tool
How to Use the Tool:
Select a job category from the dropdown menu
Choose a specific job within that category
Toggle between "Before & After" and "Training Pathways" views
Explore how the selected job is transforming and what training is needed
The tool is particularly valuable for career planning, workforce development, and organizational change management as businesses and individuals navigate the AI transformation.
Comentários